Pushing the Vanguard of Natural Language Generation
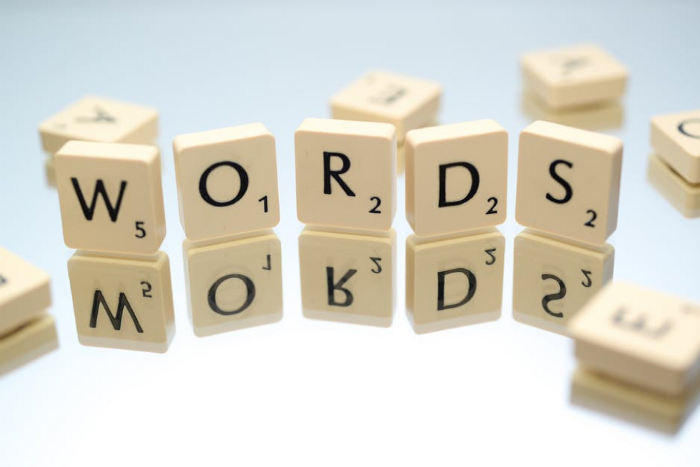
By Henry Guo & Zhiting Hu
“For last year’s words belong to last year’s language
And next year’s words await another voice.”
― T.S. Eliot, Four Quartets
These powerful and poignant words from famed poet, T.S. Eliot, capture the mood and drive of Petuum’s approach to improving artificial intelligence (AI) capabilities to preserve the beauty, the narrative, and ultimately the meaning of words through natural language generation (NLG).
NLG is one of the most exciting areas of AI research and development. Petuum and our leading team of AI experts are pushing the boundaries and looking at ways that allow for better control over various attributes of generated text (E.g. sentiment and other stylistic properties). NLG is the use of AI algorithms to generate understandable and coherent written or spoken language from a data. NLG basically translates data in many computer/machines readable form into human-ready written and spoken language. One major area of focus of NLG at Petuum is refining our ability to rapidly and accurately generate human-like, grammatically-correct, and readable text that contains all relevant information inferred from inputs.
With NLG on our minds, I want to highlight one of Petuum’s paper entries to NeurIPS 2018.
Petuum’s founder and CEO, Dr. Eric Xing, leading a varied team of researchers, including our very own Zhiting Hu, recently won submission to NeurIPS 2018, the 32nd Conference on Neural Information Processing Systems[1].
NeurIPS 2018 Paper: Unsupervised Text Style Transfer using Language Models as Discriminators
The paper titled: “Unsupervised Text Style Transfer using Language Models as Discriminators” showcases Petuum’s thought leadership on natural language generation (NLG).
The paper highlights a new technique to use a target domain language model as the discriminator for NLG. By using language models as discriminators, the team demonstrated how to outperform traditional binary classifier discriminators in three unsupervised text style transfer tasks including word substitution decipherment, sentiment modification, and related language translation.
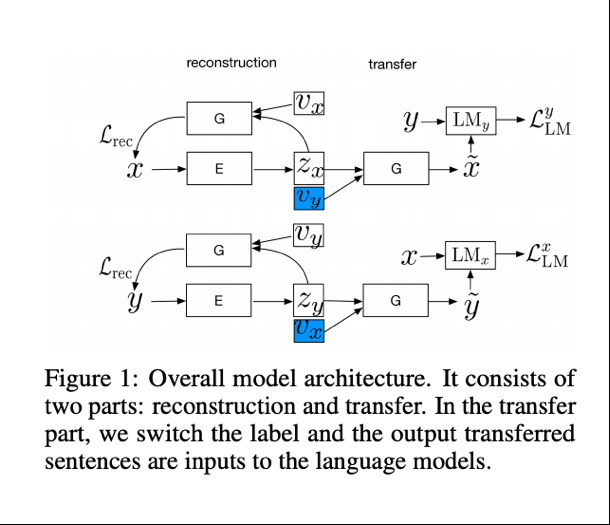
Comparison with Traditional Binary Classifier Discriminator
Previous work on unsupervised text style transfer adopts an encoder-decoder architecture with style discriminators to learn disentangled representations. The encoder takes a sentence as an input and outputs a style-independent content representation. In comparison with using a more traditional binary classifier discriminator, a language model can provide a more stable and more informative training signal for training generators. Binary classifiers are often employed as discriminators in Generative adversarial networks (GANs) -based unsupervised style transfer systems to ensure that transferred sentences are similar to sentences in the target domain. One difficulty with the binary classifier discriminator approach is that the error signal provided by the discriminator can be unstable and is sometimes insufficient to train the generator to produce fluent language.
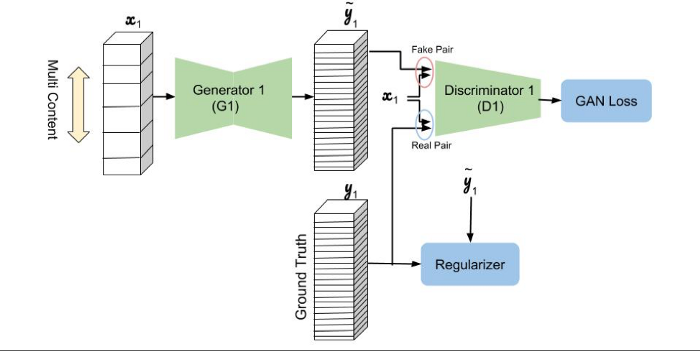
Language Model as a Structured Discriminator
By leveraging the Petuum team’s method of using a language model as a structured discriminator, it is possible to forgo adversarial steps during training, making the process more stable.
The team compared using the language model as a structured discriminator model with previous work that uses convolutional networks (CNNs) as discriminators, as well as a broad set of other approaches. Results show that the proposed method achieves improved performance on three tasks: word substitution decipherment, sentiment modification, and related language translation.
Words and language derive how we communicate, understand the world around us, learn, and share out our knowledge. By improving upon the capabilities of NLG, we look to preserving the power and meaning behind the words to help power “next year’s words” through AI.
To learn more about natural language generation we encourage you to check out our open source general-purpose AI toolkit for text generation, Texar. Read about Texar here and follow us as we continue to add updates to Texar and other areas of NLG at Petuum.
References
Zhiting Hu, Zichao Yang, Xiaodan Liang, Ruslan Salakhutdinov, and Eric P Xing. Toward controlled generation of text. ICML 2017.
Zichao Yang, Zhiting Hu, Chris Dyer, Eric P Xing, and Taylor Berg-Kirkpatrick. Unsupervised text style transfer using language models as discriminators. NIPS 2018.
Footnote: Authors of NeurIPS 2018 Paper Unsupervised Text Style Transfer using Language Models as Discriminators
Zichao Yang¹, Zhiting Hu¹, Chris Dyer², Eric P. Xing¹, Taylor Berg-Kirkpatrick¹
¹Carnegie Mellon University, ²DeepMind
{zichaoy, zhitingh, epxing, tberg}@cs.cmu.edu
cdyer@google.com
Follow us on social media to learn more about Artificial Intelligence and Machine Learning.